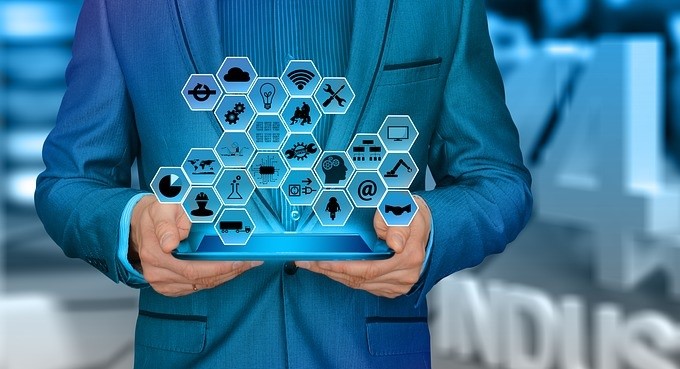
Often while shopping on eCommerce websites or App stores, we have come across the window “Recommended for you” or something similar. Recommendation Engines made those recommendations.
Recommendation Engines or Recommender System as others could call it is an application of Machine Learning technology in business to filter and make use of algorithms and data to recommend the most relevant items to a specific user. Just like the counter guy at the big boutique a few blocks away advocating one dress to you merely because he remembered you coming to buy something similar. The difference between the counter guy and the recommendation engine is that the recommendation engine is automated and more accurate and versatile.
Many commercial websites have adopted this recommender system as a marketing tool to help attractive recommend products to targeted users based on some algorithm and present data about the person or product.
What Can Recommendation Engines Do?
Although it requires some experienced programmers to set the engine up the mechanics with which it functions is comprehensible. Recommendation Engines predicts the products that a user would like and then suggests this product to the user when he/she visits the website, and this system performs this task in three possible ways: content-based suggestion, collaborative-based suggestion, and hybrid suggestion.
Content-Based Suggestion: This suggestion or recommendation is quite simple; the engine searches out products that are similar to those searched for by the user or depends on the user’s search and shopping profile to recommend products to them. For example, a buyer on an eCommerce website searches for “black leather bags,” based on this search, the recommendation engine using content-based recommendation would bring up products like black leather shoes, brown leather bags, or black bags. This is just because these are keywords searched by the user. They are also recommended based products that were previously viewed, liked or purchased by the user.
Collaborative Suggestion: The algorithm for this suggestion is a little bit more complicated. The engine recommends products based on the user’s demographics. This system collects data of people that share some level of similarities with the user and suggest products with the orientation that if two people can buy something similar before, they are likely going to buy something similar in the future. For example, shopping on Amazon.com must have brought you around the “Frequently Bought Together” window. The products on this window are collaborative suggestions based on the actions of people who have shown interest in a similar product that you are purchasing or intending to buy. So, this suggestion has little or nothing to do with the words you search for or your past shopping records instead it focuses on the activities of other users who have expressed similar interests as you.
Hybrid Suggestion: This one is a more complicating suggestion but turns out to be the most accurate. It uses both content-based and collaboration-based recommendation mechanics to recommend products to users. How is this done? Not so simple at the technical level but quite understandable at the surface level. The engine predicts products that a user would like based on the interests shared with other users and after that streamlines the predictions and recommend only those that tallies with the user’s eCommerce records and the keywords searched.
Learn many more competitive advantages for your organization by ordering Meta Leadership today.
Visit Michael Stattelman for even more….
The Essence of Recommendation Engines in Business
Recommendation engines are singular to commercial sites which are created for business purposes; and business is focused on two major things: revenue generation and customer satisfaction. The recommender system has been seen to play essential roles in achieving these focuses.
Revenue Generation: Amazon.com recorded that over 30% of their revenue is generated from recommendation sales and this has a significant impact on the business. Many people are very comfortable with recommendations from people they trust and these days; people tend to believe the internet more than they trust their fellow humans. eCommerce companies have keyed into this by providing recommendations for them on their “most trusted” internet and driving in sales as expected.
Customer Satisfaction: Most users go to eCommerce websites without a concrete decision of what to buy, after surfing their desired products, they leave the platform. Their activities are saved, and when they return, they get suggestions which appeal to them likely. They would make purchases and feel satisfied. A satisfied customer, as they say, is worth more than ten adverts.
#metaleadership